1. Cheap Parallel Computing <br> Thinking Naturally is a parallel process in which billions of neurons work simultaneously to create synchronized cortical computational waves. In order to build a neural network that is the main architecture of AI software, multiple different processes are required to be performed simultaneously. Each node of the neural network roughly represents a neuron of the brain, interacts with neighboring nodes, and understands the received signal. In order to understand a spoken word, the program must be able to hear all the relevant phonemes; in order to recognize a picture, it needs to see every pixel and surrounding pixels; these are tasks that require deep parallel computing. But until recently, the usual computer chips could only handle one task at a time.
All of this has changed since more than a decade ago, and the advent of graphics processing unit (GPU) chips can be used to meet the cumbersome visual and parallel computing needs of video games, which require multiple recalculations of millions of pixels per second. This task requires a dedicated parallel computing chip to complement the PC motherboard. This parallel graphics processing chip has worked, and the gameplay has soared. By 2005, GPU prices had fallen dramatically. In 2009, Stanford University's Andrew Ng (now joined Baidu) and his team realized that GPU chips can run neural networks in parallel.
This discovery unleashes new possibilities for neural networks, allowing the number of connections to neural network nodes to reach hundreds of millions. It takes several weeks for a traditional processor to calculate all the cascading possibilities of a 100 million-node neural network. Wu Enda found that a GPU cluster completed the same task in just one week. Today, many companies that use the cloud use a neural network that runs on top of the GPU, such as Facebook, which recognizes users' friends in photos, and provides reliable recommendations for its 50 million subscribers to Netflix.
2. Big Data <br> Every kind of intelligence needs education. Even if you are a human brain that can be classified, you need to see a dozen examples to distinguish between cats and dogs. This is especially true for artificial intelligence. Even the best-edited program requires at least a thousand games of chess to perform well. Part of the reason for the AI ​​breakthrough was the massive amount of data we collected that provided the materials needed to train the AI. Giant databases, self-tracking, web cookies, online footprints, terabytes of storage, more than a decade of search results, Wikipedia, and the entire Internet have become teachers that make AI smarter.
3. Better Algorithms <br> Digital neural networks were invented in the 1950s, but computer scientists have spent decades managing the vast mix of 1 million to 100 million neurons. The key to solving the problem is to organize the neural network by layer. For example, the relatively simple task of recognizing a human face. When a set of bits in a neural network is found to conform to a pattern (such as an eye), the result is transmitted to another layer of the neural network for further resolution. The next layer may combine the two eyes together and then transfer this meaningful result to the next layer, which in turn links the result to the nose pattern. Identifying a face may require millions of nodes (each node will generate results for use by surrounding nodes), with up to 15 layers. In 2006, Geoff Hinton, who worked at the University of Toronto, made a key improvement to this approach and named it "deep learning." He can mathematically optimize the results of each layer to speed up learning. A few years later, the deep learning algorithm was ported to the GPU, and the speed was huge. Deep learning algorithms are not enough to guarantee complex logical thinking, but it is an integral part of all current AI, including IBM's Watson, Google's search engine, and Facebook's algorithms.
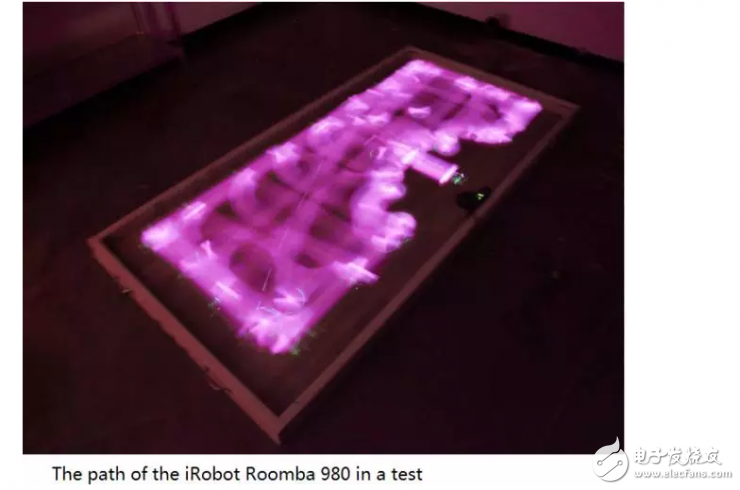
This perfect storm, consisting of parallel computing, big data and deep learning algorithms, made the 60-year AI come true overnight. And this intersection also shows that as long as these technology trends continue (and there is no reason not to continue), AI will continue to improve.
As improvements continue, this cloud-based AI will increasingly become an integral part of our daily lives. But all this has a price. Cloud computing follows the law of increasing revenue (sometimes referred to as the network effect), which means that the larger the network, the faster the growth. The larger the network, the more attractive it is to new users, which makes the network bigger, which further increases the appeal and so on. Clouds that provide AI follow the same rules. The more people use AI, the smarter AI becomes. Once a company enters this virtuous circle, it becomes bigger, grows faster, and no emerging competitor can match it. Therefore, the future AI will be ruled by two or three large general-purpose cloud AI companies.
Half man and half AI
In 1997, Watson's predecessor, Deep Blue, defeated the then chess master Garry Kasparov. After the machine won several similar games, humans basically lost interest in such games. You might think that this is the end of the story, but Kasparov realized that he could perform better if he could immediately access the massive database of the previous chess game like Dark Blue. If this database tool is fair to AI, why can't humans use it? In order to explore this idea, Kasparov took the lead in advocating the concept of human plus machine competition, that is, using AI to enhance human chess players, rather than humans against machines.
Nowadays, this type of competition is called a free-style chess game. Players can use any confrontation technique. They can move the pieces on their own or in full accordance with the requirements of the chess computer, or become "half-man AI" as advocated by Kasparov. "The player. "Half-and-a-half AI" players will listen to the chess advice provided by AI, but usually ignore this advice, just as we use GPS navigation in cars. In the 2014 Freestyle Chess Championship, the pure chess AI engine won 42 games, while the "Half-and-a-half AI" player won 53 games. The best chess player now is Intagrand, a semi-human and semi-AI, which is a team of humans and multiple chess programs.
But the most surprising thing is that the appearance of AI did not reduce the performance of pure human chess players. On the contrary, the cheap, super-smart chess program has inspired more people to play chess, the number of league games has increased, and the players have become better. The current number of chess masters is more than twice that of Deep Blue when they beat Kasparov. Today's number one human chess player, Magnus Carlsen, has been trained by AI. He is considered the most human-like human chess player and the highest ranked human chess master ever.
AI defines humans <br> If AI can help humans become better chess players, it can also help us become better pilots, doctors, referees and teachers. Most commercial work done by AI will use specialized software AI. For example, an AI can translate any language into another language, but there is nothing else in it; can drive, but can't talk; or remember YouTube. Every pixel of all videos can't predict the user's daily work. In the next 10 years, 99% of the AIs that people deal directly or indirectly will be highly specialized AIs.
In fact, this is not really smart, at least not what we think of intelligence. In fact, intelligence can be a tendency, especially if we think that "intelligence" is self-awareness. We want auto-driving cars to focus only on the road, not on the garage. Watson in the hospital should concentrate on his work, not on whether he should major in English. With the development of AI, we may want to prevent AI from generating awareness, and the most advanced AI services may advertise themselves as unconscious.
Instead, we want to be artificial intelligence rather than intelligence. Unlike the usual intelligence, wisdom is focused, measurable, and specialized. Wisdom can also think in a way that is completely different from human cognition. An interesting example of this non-human way of thinking is that IBM researchers demonstrated the use of Watson to generate recipes at the Southwest-South Conference in March this year. One of the recipes is fried fish and chips with ceviche and fried plantain. After someone tried it, I felt that the taste was not bad! Humans may not think of this recipe at all.
Non-human intelligence is not a problem, but a function. The main advantage of AI is their heterogeneous intelligence. AI thinks about food differently than chefs, which in turn allows us to think about food in different ways, thinking about making materials, clothes, thinking about financial derivatives, or science and art of any kind. The heterogeneity of artificial intelligence will be more valuable to us than its speed or power.
AI will help us understand intelligence better. In the past, we would say that super-smart AI will drive a car or defeat humans in a chess contest. And once AI does these things, we feel that these achievements are not enough to call it true intelligence. Every success achieved by AI redefines itself.
But we are not just redefining the meaning of AI, we are also redefining the meaning of human beings. Over the past 60 years, as machining has replicated what we once thought was humans only, we had to change the way we previously distinguished humans from machines. With the invention of more kinds of AI, we will be forced to give up more things that are considered to be only humans. We will face a permanent identity crisis in the next decade or century and continue to ask the meaning of human existence. The irony is that the practical benefits of everyday use AI bring to humans the greatest benefit is not to increase efficiency, bring a rich economy, or a new way of doing scientific research, but to help define humans. We need AI to tell us who it is.
As we all know that the parents all over the world pay more attention on children education, so more and more businessman do education laptop deals, therefore education laptop is becoming one of the most important fields no matter on customizing laptop or brand one. There are different series according to students ages, 14 inch celeron windows 10 education laptop for elementary students, 15.6 inch j4125 intel education laptop for middle or high school students or normal business jobs, 15.6inch 10th or 11th windows laptops for students in college or professional business or online teaching, etc. Besides, 15.6 inch 10th with 2gb graphics Programming Laptop or 16.1 inch i5 i7 i9 9th HQ GTX 1650 windows laptop for programming also available.
Other Type devices, like Yoga Laptop , Custom All In One PC, High Performance Mini PC or Android Tablet also available.
So you just need to share the education laptop price and parameters matched prefer, then right valuable information provide directly for you.
You are always welcome whatever only consult or have purchase plan recently.
Education Laptop,Education Laptop Deals,Windows 10 Education Laptop,Top Rated Student Laptops,Intel Education Laptop
Henan Shuyi Electronics Co., Ltd. , https://www.shuyilaptops.com